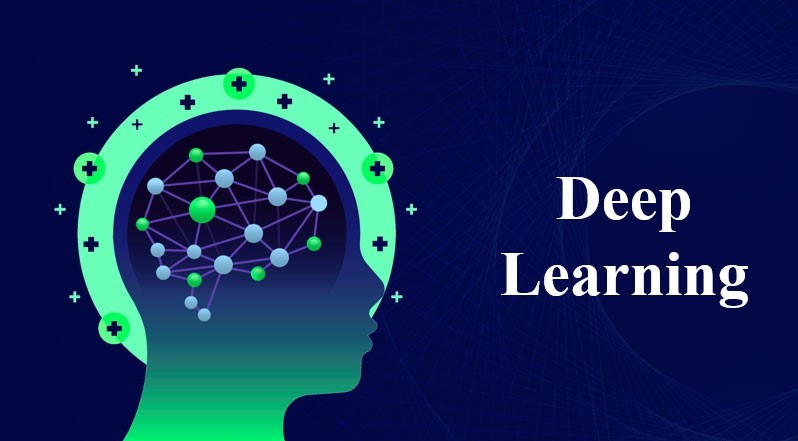
Machine Learning and AI In
Deep Learning Fundamentals
This Deep Learning Fundamentals course is designed to introduce students to the fundamental concepts and techniques of deep learning, a subset of machine learning that involves the use of artificial neural networks with multiple layers to analyze and learn from complex data. The course will cover the basics of deep learning, including neural network architectures, training methods, and applications in computer vision, natural language processing, and other areas.
Overview
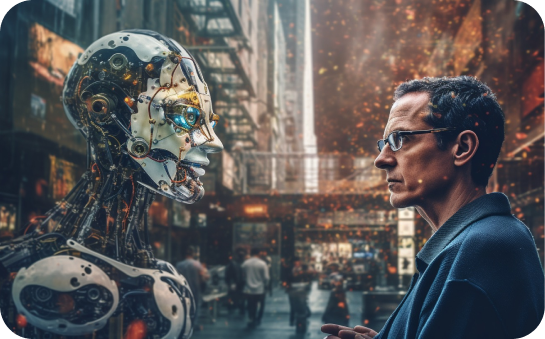
Deep Learning Fundamentals
Course Learning Outcomes (CLOs) and SLOs
Course Learning Outcomes (CLOs) typically include:
Student Learning Outcomes (SLOs) are specific goals for students, such as:
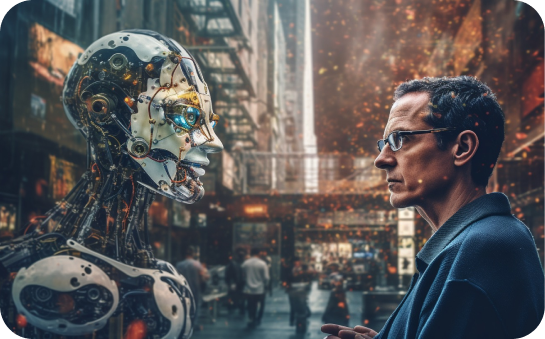
Benefits
With AI, the world is your oyster! It is an emerging field, rapidly growing, ever evolving and watched with a keen eye by industries and markets globally. There are many benefits to an education in AI:
In demand Career
With a Bachelor in artificial intelligence you are equipped with in-demand skills in the rapidly growing field of AI. Knowledge of developing AI systems, data analysis and AI techniques makes you valuable across industries, right from healthcare, finance, tech and more. This degree prepares you for career that has multiple options for diversification. AI professionals include AI engineers, data scientists, machine learning specialists, AI consultants, researchers and more. AI is transformative technology that is revolutionising the world. With an education background in AI, you are set up in an in-demand career field with an exciting future ahead!
Innovation and advancement
Applied AI is all about finding solutions and using AI systems to make life simpler. Applied AI draws on its solid foundation in Computer Science to analyse and provide solutions for real world challenges. You are prepared to address complex problems and contribute meaningfully in domains like healthcare diagnostics, fraud detection, autonomous vehicles, personalised recommendations and more. Being able to apply AI techniques for solving tasks makes for an extremely rewarding and impactful job role!
Solving real world problems
AI aims to constantly bridge the gap between natural intelligence and machine learning - it is a field of cutting edge research, innovation and advancing technology. This makes it ever evolving, with new algorithms, models and techniques being developed. By studying AI at an undergraduate level, you gain a strong foundation in AI fundamentals that help you better understand the latest advancements. You step into a career that empowers you to push the boundaries of AI, contribute to research and development and drive innovation in the field.

100% International
Study at your own pace from anywhere in the world

Recommended by 96% of our graduates
According to our latest alumni survey

50,000+ students
enrolled in Germany’s largest university
Study contents
Contents
Module 1: Introduction to Deep Learning
Key Concepts:
Module 2: Mathematical Foundations
Linear Algebra Review:
Calculus Review:
Probability Theory:
Module 3: Neural Networks
Multilayer Perceptrons (MLPs):
Convolutional Neural Networks (CNNs):
Recurrent Neural Networks (RNNs):
Module 4: Deep Learning Architectures
Autoencoders:
Generative Adversarial Networks (GANs):
Transfer Learning:
Module 5: Deep Learning Techniques
Regularization Techniques:
Optimization Algorithms:
Hyperparameter Tuning:
Module 6: Deep Learning Applications
Image Classification:
Natural Language Processing (NLP):
Speech Recognition:
Module 7: Advanced Topics in Deep Learning
Attention Mechanisms:
Batch Normalization:
Module 8: Case Studies and Projects
Real-world Applications of Deep Learning:
Module 9: Implementations and Tools
Programming Languages:Popular Deep Learning Libraries:
Data Preprocessing and Visualization Tools:
Admission
Admission Criteria
Mathematics: Calculus, Linear Algebra, Probability, and Statistics. Familiarity with mathematical concepts such as derivatives, integrals, eigenvectors, and matrix operations. Programming: Basic programming skills in a language such as Python, MATLAB, or R. Familiarity with data structures, algorithms, and software development principles. Computer Science: Understanding of computer science concepts such as data structures, algorithms, and software design patterns. Recommended Background: Linear Algebra: Understand the basics of linear algebra, including vector spaces, eigenvalues, and eigenvectors. Calculus: Familiarity with calculus concepts such as derivatives and integrals. Probability and Statistics: Understanding of basic probability theory and statistical concepts such as Bayes' theorem and hypothesis testing. Machine Learning: Familiarity with machine learning basics such as supervised and unsupervised learning, regression, classification, clustering, and dimensionality reduction. Additional Recommendations: ** Familiarity with Python**: Python is a popular language used in deep learning, so having a basic understanding of Python programming is highly recommended. Familiarity with Deep Learning Libraries: Knowledge of popular deep learning libraries such as TensorFlow, PyTorch, or Keras can be helpful but is not strictly necessary. Understanding of Data Preprocessing: Understanding of data preprocessing techniques such as data cleaning, normalization, and feature scaling can be beneficial. Course Objectives: Introduction to Deep Learning: Understand the basics of deep learning, including neural networks, convolutional neural networks (CNNs), recurrent neural networks (RNNs), long short-term memory (LSTM) networks, and autoencoders. Mathematical Foundations: Develop an understanding of the mathematical foundations of deep learning, including calculus, linear algebra, and probability theory. Deep Learning Techniques: Learn various deep learning techniques such as forward propagation, backpropagation, optimization methods, regularization techniques, and transfer learning. Hands-on Experience: Gain hands-on experience with deep learning using popular libraries such as TensorFlow or PyTorch.
Careers
Career Path
Machine Learning Engineer: Design and develop machine learning models, algorithms, and systems that enable AI-powered applications. Artificial Intelligence (AI) Researcher: Conduct research in AI and deep learning to develop new algorithms, models, and techniques for various applications. Data Scientist: Apply machine learning and deep learning techniques to analyze large datasets, identify patterns, and make predictions. Computer Vision Engineer: Develop computer vision systems that can interpret and understand visual data from images, videos, and other sources. Natural Language Processing (NLP) Engineer: Create NLP systems that can understand, generate, and process human language. Autonomous Systems Engineer: Design and develop autonomous systems that use machine learning and deep learning for self-driving cars, drones, and other applications. Robotics Engineer: Apply machine learning and deep learning to develop intelligent robots that can perceive, reason, and interact with their environment. Quantum Computing Researcher: Explore the application of machine learning and deep learning to quantum computing and its potential for solving complex problems. Image Processing Specialist: Develop image processing algorithms using deep learning techniques for applications such as medical imaging, satellite imaging, or surveillance. Speech Recognition Engineer: Create speech recognition systems that use machine learning and deep learning to recognize and transcribe spoken language. Recommendation Systems Engineer: Design and develop recommendation systems that use machine learning and deep learning to suggest products or services based on user behavior. Bioinformatics Researcher: Apply machine learning and deep learning to analyze large biological datasets, such as genomic sequences or medical imaging data. Finance Data Scientist: Use machine learning and deep learning to analyze financial data, predict market trends, and make investment decisions. Marketing Analyst: Apply machine learning and deep learning to analyze customer behavior, optimize marketing campaigns, and predict customer churn. Healthcare Data Analyst: Use machine learning and deep learning to analyze healthcare data, predict patient outcomes, and develop personalized treatment plans.
Student reviews
Coming Soon.
Tuition fees
All our study programmes include the following benefits
- Teaching and study material
- Marking of your end-of-module exams
- Monthly live and recorded tutorials
- Use of the online campus
- Individual study coaching
- Online exams
- Career coaching
- Learn English for free
Our global recognition
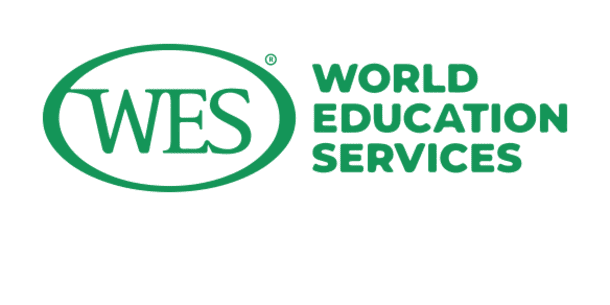
IU is recognised by WES Canada and U.S., which means your degree can be converted to points in the local system for purposes of immigration, work, or studies.
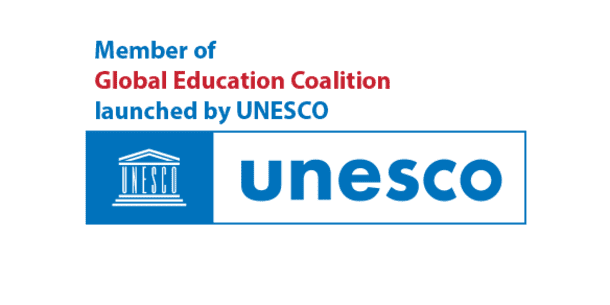
As the first EU institution in UNESCO's Global Education Coalition, IU is committed to ensuring accessible quality education to students in crisis worldwide through free online micro-credentials.
Our company partners
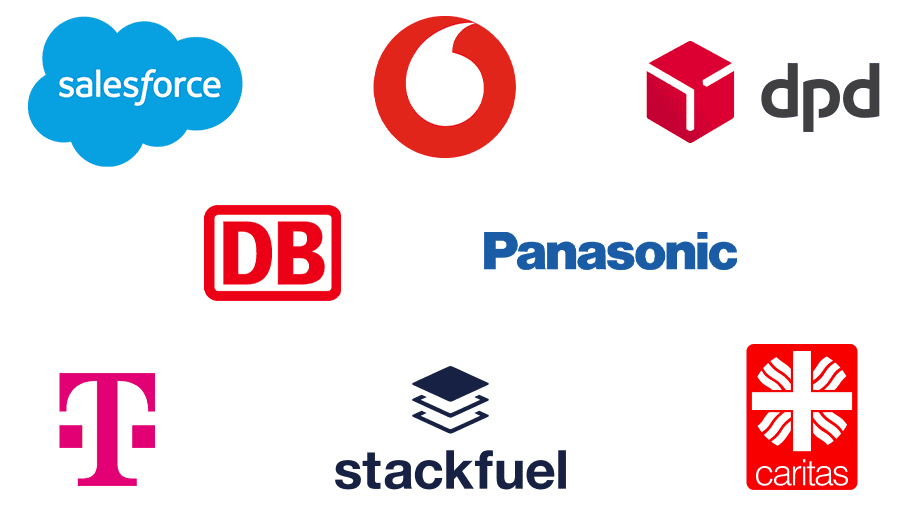
For over 20 years, IU has established partnerships with leading global companies. This offers you the chance to gain firsthand experience through internships and projects and allow us to adapt our learning content to the ever-evolving needs of the labour market. You'll benefit from an education designed to bridge the gap between theory and real-world practice, ensuring your readiness for your future career.
Recognition
Recognition of previous achievements
Have you already completed a training course, studied at a university or gained work experience? Have you completed a course or a learning path through EPIBM LinkedIn Learning, and earned a certificate? Then you have the opportunity to get your previous achievements recognised, and complete your studies at EPIBM sooner.
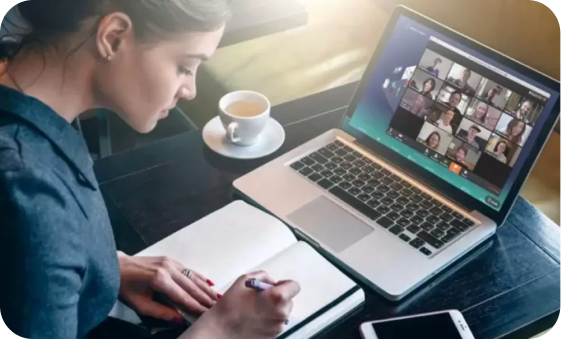
Save time:
Skip individual modules or whole semesters!
Even before you apply for a study programme, we’ll gladly check whether we can take your previous achievements into account: 100% online, no strings attached. Simply fill in our recognition application form, which you can find under the content section of each study programme's webpage, and upload it via our upload section. You can also e-mail it to us, or send it via post.
Send an email to [email protected] to find out which previous achievements you can get recognised. You can get your previous achievements recognised during your studies.
Recognition files
Autonomous vehicles developer
With AI, the world is your oyster! It is an emerging field, rapidly growing, ever evolving and watched with a keen eye by industries and markets globally. There are many benefits to an education in AI:
That’s why after graduating, you’ll be able to apply your professional skills and knowledge, and work for development teams at any sector you find appealing.
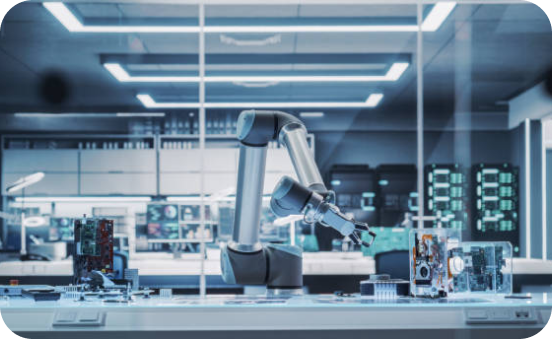
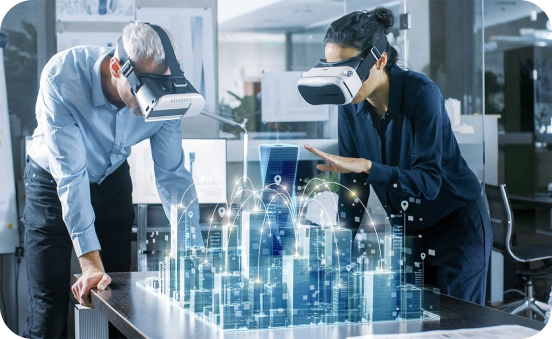
Augmented reality (AR/VR) developer
Virtual (or augmented) reality isn’t all just fun and games, as great and enjoyable as that aspect is. It can also be used for groundbreaking social and psychological research, defensive purposes and therapy.
With an Applied Artificial Intelligence degree from IU University of Applied Sciences, you can take part in this vital field of technological development, and work on a wide variety of interesting projects.
Change what the world thinks about the possibilities that AI offers, and make a real difference in people’s lives, while enjoying every step of the process.
F.A.Q
Frequently Asked Questions
You might also be interested in these study programmes
Accredited and certified