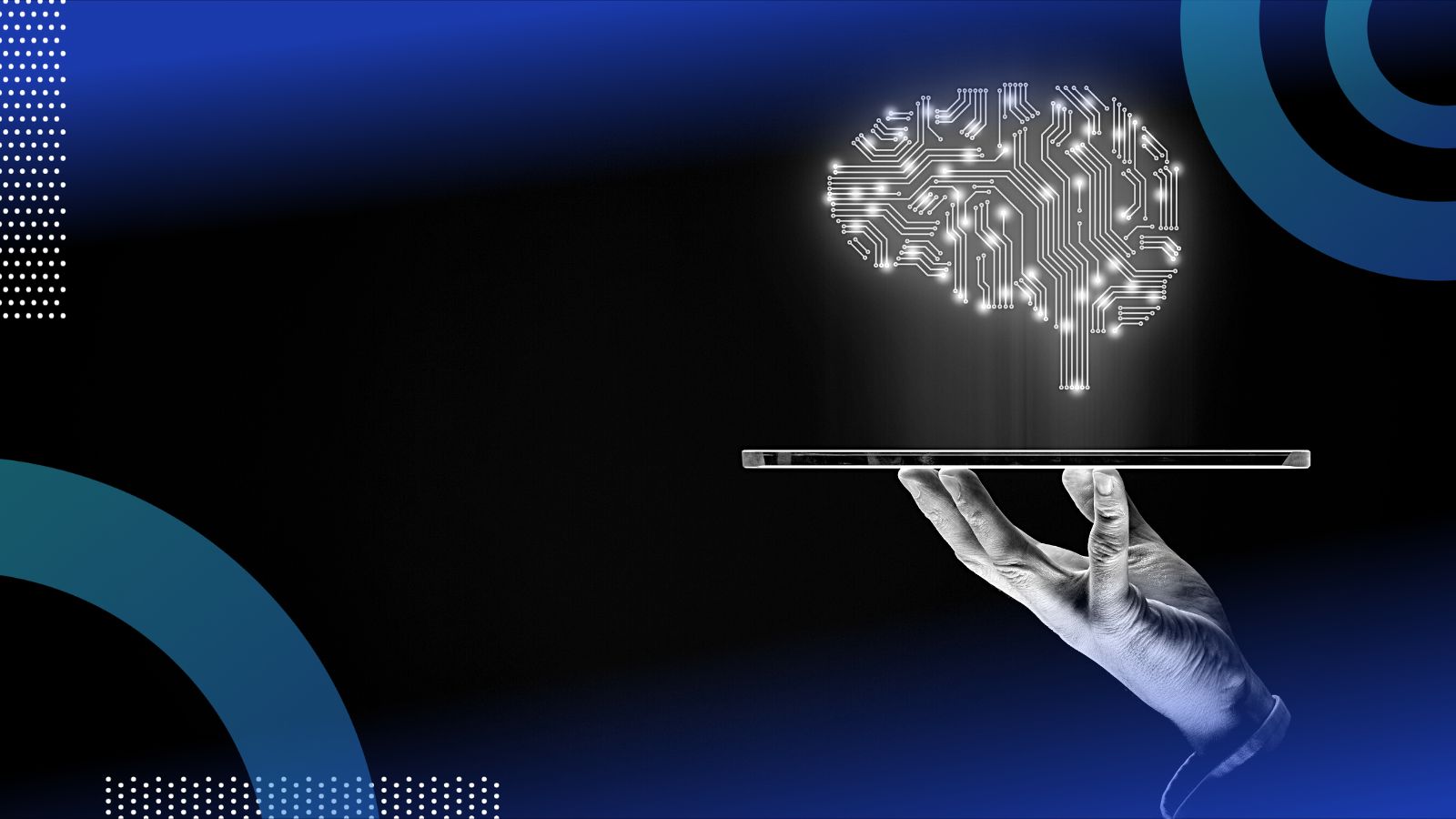
Machine Learning and AI In
Machine Learning Basics
This Machine Learning Basics course is designed to introduce students to the fundamental concepts of machine learning, including supervised and unsupervised learning, regression, classification, clustering, and neural networks. Students will learn how to implement machine learning algorithms using popular libraries such as scikit-learn and TensorFlow.
Overview
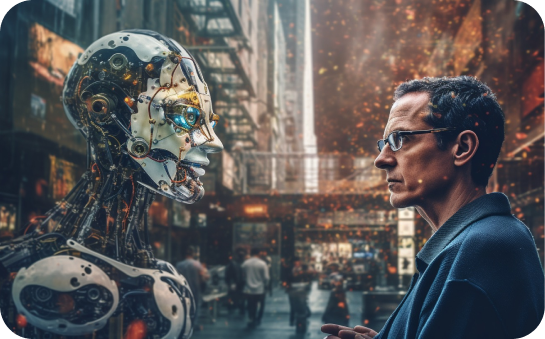
Machine Learning Basics
Course Learning Outcomes (CLOs) and SLOs
Course Learning Outcomes (CLOs) typically include:
Student Learning Outcomes (SLOs) are specific goals for students, such as:
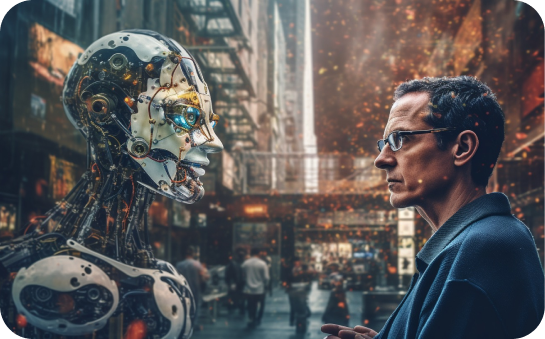
Benefits
With AI, the world is your oyster! It is an emerging field, rapidly growing, ever evolving and watched with a keen eye by industries and markets globally. There are many benefits to an education in AI:
In demand Career
With a Bachelor in artificial intelligence you are equipped with in-demand skills in the rapidly growing field of AI. Knowledge of developing AI systems, data analysis and AI techniques makes you valuable across industries, right from healthcare, finance, tech and more. This degree prepares you for career that has multiple options for diversification. AI professionals include AI engineers, data scientists, machine learning specialists, AI consultants, researchers and more. AI is transformative technology that is revolutionising the world. With an education background in AI, you are set up in an in-demand career field with an exciting future ahead!
Innovation and advancement
Applied AI is all about finding solutions and using AI systems to make life simpler. Applied AI draws on its solid foundation in Computer Science to analyse and provide solutions for real world challenges. You are prepared to address complex problems and contribute meaningfully in domains like healthcare diagnostics, fraud detection, autonomous vehicles, personalised recommendations and more. Being able to apply AI techniques for solving tasks makes for an extremely rewarding and impactful job role!
Solving real world problems
AI aims to constantly bridge the gap between natural intelligence and machine learning - it is a field of cutting edge research, innovation and advancing technology. This makes it ever evolving, with new algorithms, models and techniques being developed. By studying AI at an undergraduate level, you gain a strong foundation in AI fundamentals that help you better understand the latest advancements. You step into a career that empowers you to push the boundaries of AI, contribute to research and development and drive innovation in the field.

100% International
Study at your own pace from anywhere in the world

Recommended by 96% of our graduates
According to our latest alumni survey

50,000+ students
enrolled in Germany’s largest university
Study contents
Contents
Section 1: Introduction to Machine Learning
Section 2: Supervised Learning
Section 3: Unsupervised Learning
Section 4: Reinforcement Learning
Section 5: Neural Networks
Section 6: Deep Learning
Section 7: Model Evaluation and Selection
Section 8: Machine Learning Algorithms
Section 9: Advanced Topics
Section 10: Real-World Applications
Admission
Admission Criteria
Prerequisites: Basic understanding of programming concepts (Python, R, or any other programming language) Familiarity with mathematics and statistics (linear algebra, calculus, probability) Experience with data analysis and visualization tools (e.g., Pandas, NumPy, Matplotlib) Admission Process: Step 1: Understand the Basics of Machine Learning Learn about supervised and unsupervised learning, regression, classification, clustering, and neural networks Familiarize yourself with popular machine learning algorithms (e.g., Linear Regression, Decision Trees, Random Forests) Study the concept of bias-variance tradeoff and overfitting Step 2: Choose a Programming Language Select a programming language for machine learning (Python is a popular choice) Install necessary libraries and tools (e.g., scikit-learn, TensorFlow, Keras) Step 3: Get Familiar with Data Preprocessing Learn about data preprocessing techniques (e.g., data cleaning, feature scaling, feature selection) Understand the importance of data quality and handling missing values Practice working with datasets to prepare them for machine learning models Step 4: Learn Model Evaluation and Selection Understand how to evaluate the performance of machine learning models (e.g., accuracy, precision, recall, F1-score) Learn how to compare and select the best model for a given problem Study techniques for hyperparameter tuning and model selection Step 5: Practice with Real-World Datasets Find publicly available datasets (e.g., UCI Machine Learning Repository, Kaggle Datasets) Practice implementing machine learning models on real-world datasets Experiment with different algorithms and hyperparameters to improve model performance Step 6: Learn Advanced Topics Study advanced topics in machine learning (e.g., deep learning, natural language processing, computer vision) Learn about state-of-the-art techniques and recent advancements in the field Step 7: Join Online Communities and Take Online Courses Join online communities (e.g., Kaggle, Reddit's r/MachineLearning) to stay updated with the latest developments Take online courses or attend webinars to further your knowledge and skills
Careers
Career Path
Data Scientist: Work with large datasets to identify patterns, build predictive models, and make data-driven decisions. Machine Learning Engineer: Design, develop, and deploy machine learning models for various applications, such as natural language processing, computer vision, or recommender systems. Artificial Intelligence (AI) Researcher: Conduct research in AI and machine learning to develop new algorithms, techniques, and models that can be applied to various fields. Predictive Modeling Specialist: Build predictive models using machine learning to forecast outcomes, identify trends, and make informed decisions. Business Analyst: Apply machine learning techniques to analyze business data and make data-driven recommendations to improve business operations. Computer Vision Engineer: Work on image and video analysis, object detection, and recognition using machine learning algorithms. Natural Language Processing (NLP) Specialist: Develop NLP models for text analysis, sentiment analysis, language translation, and chatbots. Recommendation System Developer: Design and implement personalized recommendation systems for e-commerce, social media, or other applications. Automated Decision Systems (ADS) Developer: Build systems that use machine learning to automate decision-making processes in areas like finance, healthcare, or marketing. Quantitative Analyst: Apply machine learning techniques to financial modeling, risk analysis, and portfolio optimization in finance. Operations Research Analyst: Use machine learning and optimization techniques to improve operational efficiency in industries like logistics, supply chain management, or energy management. Healthcare Data Analyst: Analyze healthcare data using machine learning to identify trends, predict patient outcomes, and improve treatment plans. Marketing Analyst: Use machine learning to analyze customer behavior, predict consumer preferences, and optimize marketing campaigns. Risk Analysis Specialist: Apply machine learning to identify and mitigate risks in areas like finance, cybersecurity, or environmental sustainability. Education Technology (EdTech) Specialist: Develop educational software using machine learning to personalize learning experiences for students. Digital Forensics Analyst: Use machine learning to analyze digital evidence in criminal investigations and cybersecurity incidents. Robotics Engineer: Combine machine learning with robotics to develop intelligent robots that can perceive their environment and interact with humans. Game Developer: Apply machine learning to create more realistic game environments, NPCs (non-player characters), and game mechanics. Cybersecurity Specialist: Use machine learning to detect and prevent cyber attacks by analyzing network traffic patterns and identifying anomalies. Academic Researcher: Pursue a career in academia, conducting research in machine learning and its applications in various fields.
Student reviews
Coming Soon.
Tuition fees
Machine Learning Basics (Duration 4 Weeks)
This opens up chances for Machine Learning engineers, data scientists, data mining specialists, and data engineers in various industries. Therefore, if you aspire to be among the industry's most valuable professionals, you must learn machine learning.
350 $
200 $ / Total CostAll our study programmes include the following benefits
- Teaching and study material
- Marking of your end-of-module exams
- Monthly live and recorded tutorials
- Use of the online campus
- Individual study coaching
- Online exams
- Career coaching
- Learn English for free
Our global recognition
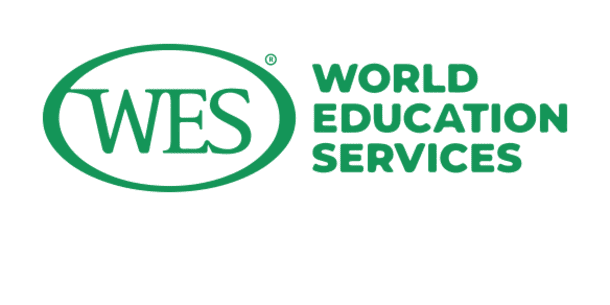
IU is recognised by WES Canada and U.S., which means your degree can be converted to points in the local system for purposes of immigration, work, or studies.
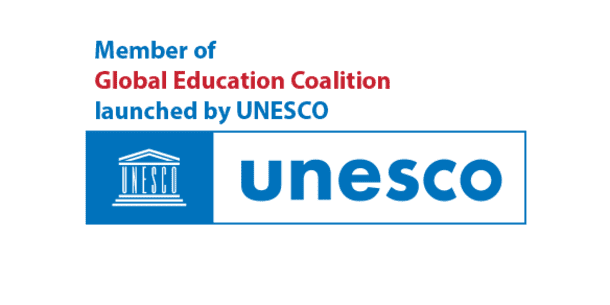
As the first EU institution in UNESCO's Global Education Coalition, IU is committed to ensuring accessible quality education to students in crisis worldwide through free online micro-credentials.
Our company partners
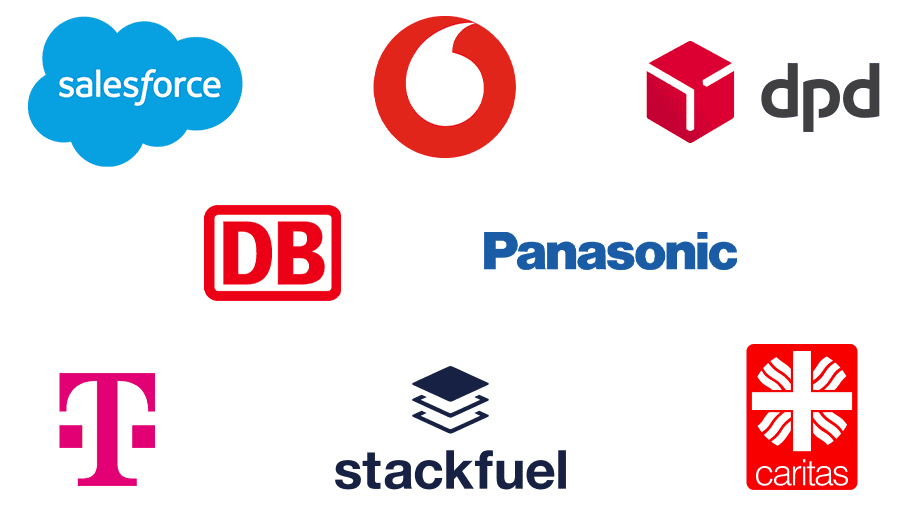
For over 20 years, IU has established partnerships with leading global companies. This offers you the chance to gain firsthand experience through internships and projects and allow us to adapt our learning content to the ever-evolving needs of the labour market. You'll benefit from an education designed to bridge the gap between theory and real-world practice, ensuring your readiness for your future career.
Recognition
Recognition of previous achievements
Have you already completed a training course, studied at a university or gained work experience? Have you completed a course or a learning path through EPIBM LinkedIn Learning, and earned a certificate? Then you have the opportunity to get your previous achievements recognised, and complete your studies at EPIBM sooner.
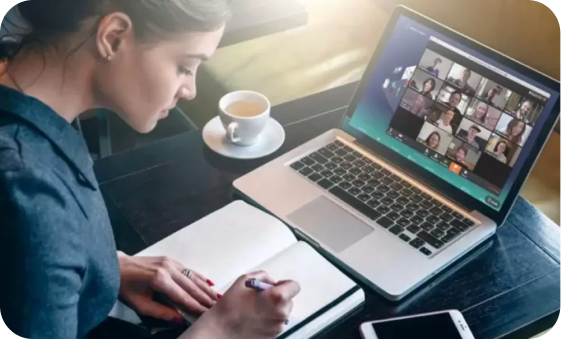
Save time:
Skip individual modules or whole semesters!
Even before you apply for a study programme, we’ll gladly check whether we can take your previous achievements into account: 100% online, no strings attached. Simply fill in our recognition application form, which you can find under the content section of each study programme's webpage, and upload it via our upload section. You can also e-mail it to us, or send it via post.
Send an email to [email protected] to find out which previous achievements you can get recognised. You can get your previous achievements recognised during your studies.
Recognition files
Autonomous vehicles developer
With AI, the world is your oyster! It is an emerging field, rapidly growing, ever evolving and watched with a keen eye by industries and markets globally. There are many benefits to an education in AI:
That’s why after graduating, you’ll be able to apply your professional skills and knowledge, and work for development teams at any sector you find appealing.
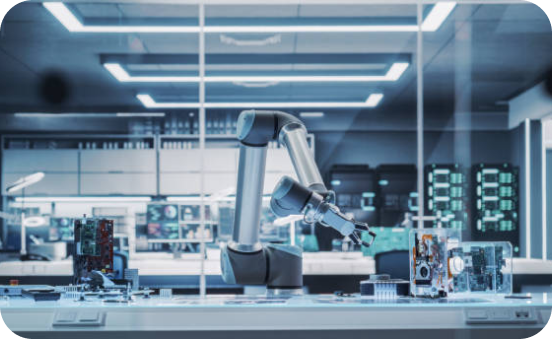
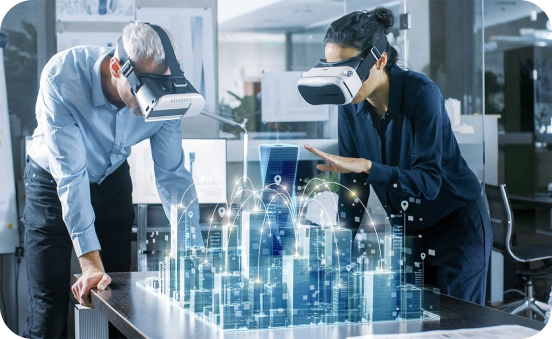
Augmented reality (AR/VR) developer
Virtual (or augmented) reality isn’t all just fun and games, as great and enjoyable as that aspect is. It can also be used for groundbreaking social and psychological research, defensive purposes and therapy.
With an Applied Artificial Intelligence degree from IU University of Applied Sciences, you can take part in this vital field of technological development, and work on a wide variety of interesting projects.
Change what the world thinks about the possibilities that AI offers, and make a real difference in people’s lives, while enjoying every step of the process.
F.A.Q
Frequently Asked Questions
You might also be interested in these study programmes
Accredited and certified