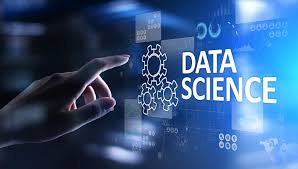
Data Science and Analytics In
Introduction to Data Science
This Introduction to Data Science course is designed to introduce students to the fundamentals of data science, including data analysis, visualization, and machine learning. The course will cover the entire data science workflow, from data wrangling and cleaning to data visualization and modeling. Students will learn how to extract insights from large datasets using Python programming language and popular data science libraries.
Overview
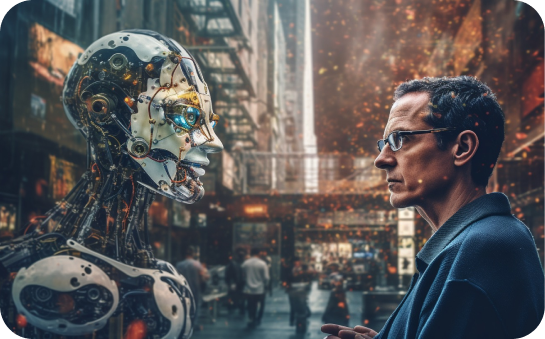
Introduction to Data Science
Course Learning Outcomes (CLOs) and SLOs
Course Learning Outcomes (CLOs) typically include:
Data Manipulation: Gain proficiency in manipulating and cleaning data using programming languages such as Python or R.
Statistical Analysis: Understand basic statistical methods and techniques used for analyzing data and drawing meaningful conclusions.
Data Visualization: Learn to visualize data effectively using graphs, charts, and other visualization techniques to communicate insights.
Machine Learning Concepts: Acquire knowledge of fundamental machine learning concepts and algorithms for predictive analytics.
Student Learning Outcomes (SLOs) are specific goals for students, such as:
Data Analysis Skills: Apply data analysis techniques to extract useful information and patterns from datasets.
Programming Proficiency: Demonstrate proficiency in using programming languages (e.g., Python, R) for data manipulation, analysis, and visualization.
Critical Thinking: Develop critical thinking skills to evaluate data and make informed decisions based on analysis results.
Project Execution: Successfully execute a data science project, from data acquisition and cleaning to analysis and presentation of findings.
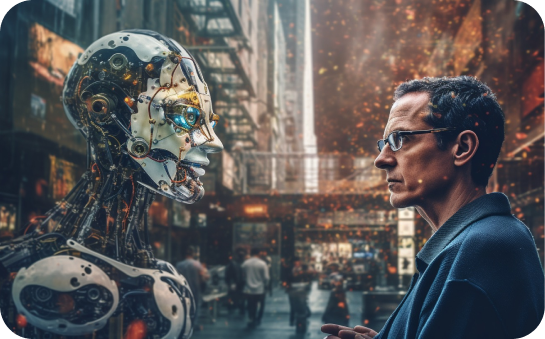
Benefits
With AI, the world is your oyster! It is an emerging field, rapidly growing, ever evolving and watched with a keen eye by industries and markets globally. There are many benefits to an education in AI:
In demand Career
With a Bachelor in artificial intelligence you are equipped with in-demand skills in the rapidly growing field of AI. Knowledge of developing AI systems, data analysis and AI techniques makes you valuable across industries, right from healthcare, finance, tech and more. This degree prepares you for career that has multiple options for diversification. AI professionals include AI engineers, data scientists, machine learning specialists, AI consultants, researchers and more. AI is transformative technology that is revolutionising the world. With an education background in AI, you are set up in an in-demand career field with an exciting future ahead!
Innovation and advancement
Applied AI is all about finding solutions and using AI systems to make life simpler. Applied AI draws on its solid foundation in Computer Science to analyse and provide solutions for real world challenges. You are prepared to address complex problems and contribute meaningfully in domains like healthcare diagnostics, fraud detection, autonomous vehicles, personalised recommendations and more. Being able to apply AI techniques for solving tasks makes for an extremely rewarding and impactful job role!
Solving real world problems
AI aims to constantly bridge the gap between natural intelligence and machine learning - it is a field of cutting edge research, innovation and advancing technology. This makes it ever evolving, with new algorithms, models and techniques being developed. By studying AI at an undergraduate level, you gain a strong foundation in AI fundamentals that help you better understand the latest advancements. You step into a career that empowers you to push the boundaries of AI, contribute to research and development and drive innovation in the field.

100% International
Study at your own pace from anywhere in the world

Recommended by 96% of our graduates
According to our latest alumni survey

50,000+ students
enrolled in Germany’s largest university
Study contents
Contents
Module 1: What is Data Science?
Definition and scope of data science
Brief history of data science
Key concepts: data, information, knowledge, and wisdom
Importance of data science in today's world
Module 2: Types of Data
Descriptive statistics
Inferential statistics
Visualizing data with plots and charts
Categorical and numerical data types
Handling missing data
Module 3: Data Preprocessing and Cleaning
Data wrangling: handling missing values, outliers, and duplicatesv
Data quality control: detecting errors and inconsistencies
Data transformation: normalization, aggregation, and feature engineering
Handling imbalanced datasets
Module 4: Machine Learning Fundamentals
Introduction to supervised and unsupervised learning
Regression, classification, clustering, and dimensionality reduction algorithms
Model evaluation metrics: accuracy, precision, recall, F1-score, and ROC-AUC
Overfitting and underfitting
Module 5: Data Mining and Visualization
Association rule mining
Decision trees and random forests
Clustering algorithms (k-means, hierarchical)
Dimensionality reduction techniques (PCA, t-SNE)
Data visualization tools (Matplotlib, Seaborn)
Module 6: Big Data and NoSQL Databases
Introduction to big data and Hadoop ecosystem
HDFS (Hadoop Distributed File System)
MapReduce programming model
NoSQL databases (HBase, Cassandra, MongoDB)
Module 7: Deep Learning and Neural Networks
Introduction to neural networks and deep learning
Basic concepts: neurons, layers, activation functions, backpropagation
Deep learning frameworks (TensorFlow, PyTorch)
Applications of deep learning in computer vision, natural language processing
Module 8: Case Studies and Projects
Real-world case studies in various industries (healthcare, finance, marketing)
Group projects on applying data science concepts to real-world problems
Presentations and feedback from peers and instructors
Module 9: Ethical Considerations in Data Science
Ethics in data collection and storage
Data privacy and security concerns
Bias in machine learning models
Responsible data sharing and open data initiatives
Admission
Admission Criteria
General Requirements:
Academic Background: Most Introduction to Data Science courses are designed for students with a basic understanding of mathematics and statistics, typically requiring a high school diploma or equivalent.
Computer Skills: Familiarity with computers and basic programming concepts (e.g., loops, variables, data structures) is often assumed.
Math Skills: A basic understanding of algebra, geometry, and probability is usually required.
Specific Course-Specific Requirements:
Prerequisites: Some courses may have specific prerequisites, such as:
Linear Algebra
Statistics
Programming (e.g., Python, R)
Math Calculus
Language Proficiency: Depending on the course, proficiency in a programming language like Python, R, or SQL may be required.
Additional Requirements: Some courses may require additional materials or tools, such as:
A laptop with a specific operating system (e.g., Windows, macOS)
Specialized software (e.g., Tableau, Power BI)
Online accounts or subscriptions (e.g., Kaggle, Coursera)
Careers
Career Path
Data Scientist: A data scientist is responsible for extracting insights from large datasets to inform business decisions. They design and implement data models, algorithms, and visualizations to analyze complex data.
Data Analyst: A data analyst is a more junior role that focuses on extracting insights from data to support business decision-making. They typically work with smaller datasets and focus on descriptive analytics.
Business Intelligence Developer: A business intelligence developer designs and implements data visualizations, reports, and dashboards to help organizations make better decisions.
Data Engineer: A data engineer is responsible for designing and building the infrastructure to store, process, and retrieve large datasets.
Machine Learning Engineer: A machine learning engineer designs and develops predictive models using machine learning algorithms to solve complex problems.
Data Architect: A data architect designs the overall data strategy and infrastructure for an organization, ensuring that data is stored, processed, and retrieved efficiently.
Statistical Analyst: A statistical analyst applies statistical techniques to extract insights from data and make recommendations for business decisions.
Quantitative Analyst: A quantitative analyst uses mathematical and statistical techniques to analyze financial data and make investment recommendations.
Operations Research Analyst: An operations research analyst uses advanced analytics to optimize business processes and solve complex problems.
Database Administrator: A database administrator manages and maintains the organization's databases, ensuring data integrity and security.
Student reviews
Coming Soon.
Tuition fees
All our study programmes include the following benefits
- Teaching and study material
- Marking of your end-of-module exams
- Monthly live and recorded tutorials
- Use of the online campus
- Individual study coaching
- Online exams
- Career coaching
- Learn English for free
Our global recognition
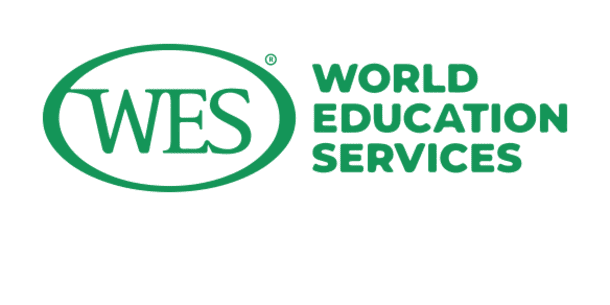
IU is recognised by WES Canada and U.S., which means your degree can be converted to points in the local system for purposes of immigration, work, or studies.
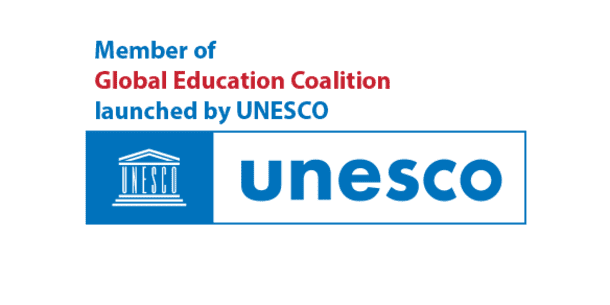
As the first EU institution in UNESCO's Global Education Coalition, IU is committed to ensuring accessible quality education to students in crisis worldwide through free online micro-credentials.
Our company partners
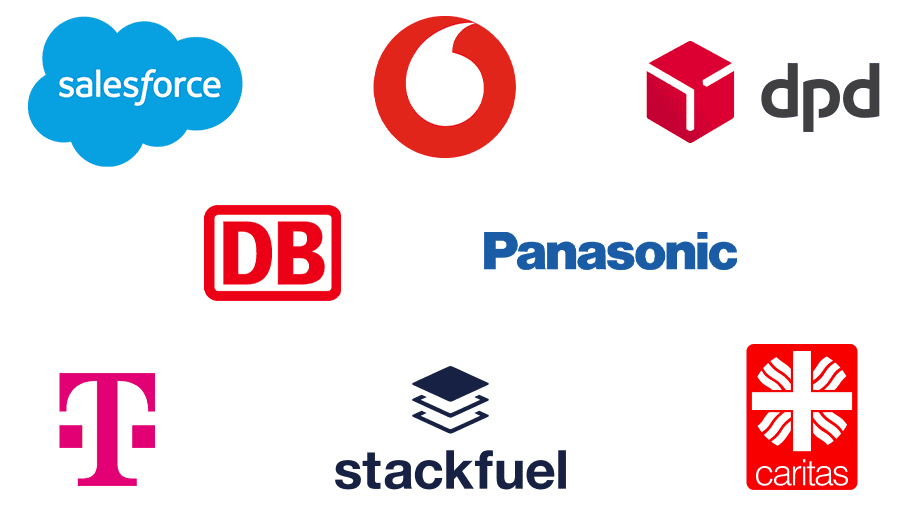
For over 20 years, IU has established partnerships with leading global companies. This offers you the chance to gain firsthand experience through internships and projects and allow us to adapt our learning content to the ever-evolving needs of the labour market. You'll benefit from an education designed to bridge the gap between theory and real-world practice, ensuring your readiness for your future career.
Recognition
Recognition of previous achievements
Have you already completed a training course, studied at a university or gained work experience? Have you completed a course or a learning path through EPIBM LinkedIn Learning, and earned a certificate? Then you have the opportunity to get your previous achievements recognised, and complete your studies at EPIBM sooner.
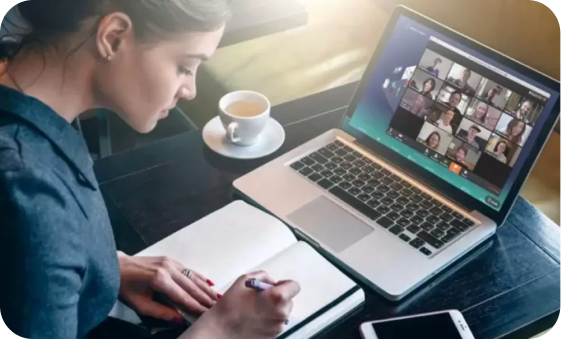
Save time:
Skip individual modules or whole semesters!
Even before you apply for a study programme, we’ll gladly check whether we can take your previous achievements into account: 100% online, no strings attached. Simply fill in our recognition application form, which you can find under the content section of each study programme's webpage, and upload it via our upload section. You can also e-mail it to us, or send it via post.
Send an email to epibm.uk@gmail.com to find out which previous achievements you can get recognised. You can get your previous achievements recognised during your studies.
Recognition files
Autonomous vehicles developer
With AI, the world is your oyster! It is an emerging field, rapidly growing, ever evolving and watched with a keen eye by industries and markets globally. There are many benefits to an education in AI:
That’s why after graduating, you’ll be able to apply your professional skills and knowledge, and work for development teams at any sector you find appealing.
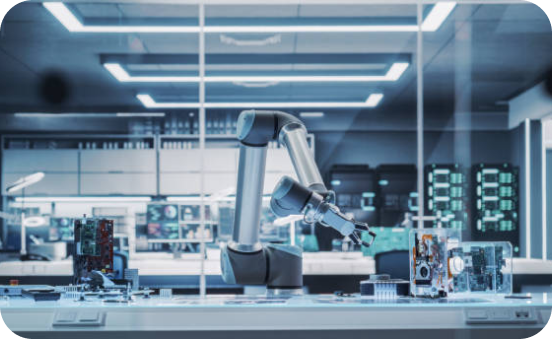
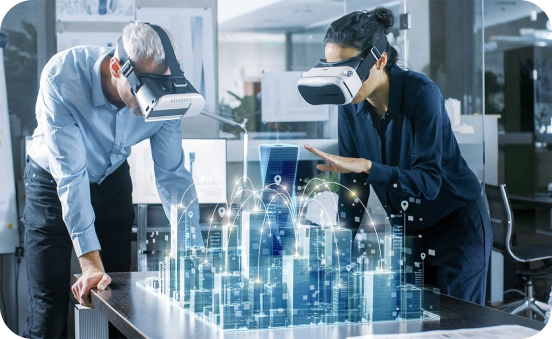
Augmented reality (AR/VR) developer
Virtual (or augmented) reality isn’t all just fun and games, as great and enjoyable as that aspect is. It can also be used for groundbreaking social and psychological research, defensive purposes and therapy.
With an Applied Artificial Intelligence degree from IU University of Applied Sciences, you can take part in this vital field of technological development, and work on a wide variety of interesting projects.
Change what the world thinks about the possibilities that AI offers, and make a real difference in people’s lives, while enjoying every step of the process.
F.A.Q
Frequently Asked Questions
You might also be interested in these study programmes
Accredited and certified